As artificial intelligence (AI) continues to advance, its applications in decision-making, automation, and personalization are becoming indispensable across industries. However, with this growing influence comes a pressing need to ensure AI systems are ethically designed and implemented. Ethical AI is not only a technical requirement but also a societal demand, as trust in AI systems is crucial for their widespread acceptance. This article delves into the core principles of AI ethics, explores challenges, and provides strategies for building trust with AI solutions.
Core Principles of AI Ethics
Transparency
Transparency is the cornerstone of ethical AI. It ensures that the decision-making processes of AI systems are understandable and explainable to users and stakeholders. Open-source AI models and clear documentation are critical for achieving transparency. For instance, explainable AI (XAI) frameworks enable organizations to provide insights into how their algorithms reach conclusions, fostering trust.
Fairness
AI systems must operate without bias, ensuring fairness in outcomes regardless of users’ demographics or circumstances. Bias often originates from skewed training data or poorly designed algorithms. Techniques such as algorithmic fairness assessments and diverse training datasets are essential to mitigate bias and promote inclusivity.
Accountability
Assigning responsibility for AI decisions is essential for ethical implementation. Organizations must establish clear accountability structures, ensuring that both developers and deployers take ownership of AI outcomes. Regulatory frameworks like the EU AI Act provide guidance on maintaining accountability in AI systems.
Privacy
AI ethics prioritizes safeguarding user data. With AI systems processing vast amounts of sensitive information, robust encryption methods and data anonymization techniques are critical. Balancing data utility and privacy remains a challenge, necessitating thoughtful design and adherence to privacy laws such as GDPR.
Safety
AI solutions must prioritize safety, minimizing potential harm to users and systems. This includes rigorous testing for unintended consequences and building fail-safe mechanisms. Safety measures are particularly crucial in high-stakes applications like healthcare and autonomous vehicles.
Key Ethical Challenges in AI
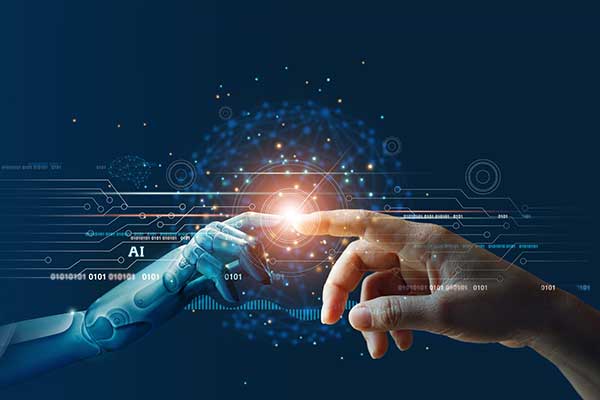
Bias in AI Systems
Bias remains one of the most significant challenges in AI. For example, facial recognition algorithms have been criticized for their inaccuracies in identifying individuals from minority groups. Addressing bias requires diverse training data and continuous algorithm evaluations.
Lack of Transparency
Many AI systems operate as “black boxes,” making it difficult to understand their decision-making processes. This lack of transparency can erode trust. Techniques like model interpretability and explainable frameworks can bridge this gap.
Data Privacy Concerns
AI systems often require extensive data for training and operation. This creates ethical dilemmas around data collection, consent, and usage. Businesses must adopt transparent data policies and prioritize user consent to build trust.
Misuse of AI
The potential misuse of AI for surveillance, weaponization, or spreading misinformation poses ethical risks. Limiting the use of AI in harmful applications and establishing global guidelines can mitigate these threats.
Dependency and Job Displacement
AI automation has raised concerns about job displacement and dependency on technology. Ethical AI development must include strategies to retrain affected workers and ensure equitable access to AI benefits.
Strategies for Building Trust in AI Solutions
Ethical AI Design and Development
Incorporating ethical considerations during the design phase is crucial. This includes conducting ethical impact assessments and embedding fairness, accountability, and safety into the AI lifecycle. Tools like IBM’s AI Fairness 360 toolkit help developers create ethical solutions.
Stakeholder Involvement
Engaging diverse stakeholders—including developers, policymakers, and end-users—ensures that AI systems address real-world ethical concerns. Multidisciplinary teams bring varied perspectives, enriching the ethical development process.
Regulatory and Compliance Frameworks
Governments and organizations worldwide are establishing guidelines for ethical AI. Frameworks such as the IEEE Global Initiative on Ethics of Autonomous and Intelligent Systems and the EU AI Act provide actionable standards for developers.
Transparency and Communication
AI developers must communicate openly about their systems’ capabilities and limitations. User-friendly explainability features and clear documentation build confidence in AI solutions.
Continuous Monitoring and Improvement
Ethical AI is an ongoing commitment. Regular audits, real-time monitoring, and user feedback loops ensure that AI systems remain fair, transparent, and effective.
Case Studies in AI Ethics
Positive Examples
- Google’s AI Principles: Google’s commitment to ethical AI includes principles such as avoiding bias, prioritizing safety, and advancing beneficial AI applications.
- Microsoft’s Responsible AI Program: This program emphasizes transparency, fairness, and accountability in AI systems, earning user trust.
Lessons from Ethical Failures
- Discriminatory Hiring Algorithms: An AI hiring tool was found to favor male candidates due to biased training data. This highlights the importance of diverse and balanced datasets.
- Biased Facial Recognition: High-profile incidents of racial bias in facial recognition systems have underscored the need for rigorous testing and unbiased datasets.
The Role of Ethical AI in Building Trust
Trust as a Foundation for AI Adoption
Ethical AI practices are essential for gaining public confidence and ensuring long-term adoption. Transparent, fair, and accountable systems are more likely to be embraced by users.
Ethical AI and Brand Reputation
Companies that prioritize ethics in AI development enhance their brand reputation. Ethical practices demonstrate corporate responsibility and align with consumer expectations.
Long-Term Implications of Ethical AI
Investing in ethical AI fosters a sustainable AI ecosystem, paving the way for innovations that benefit society while minimizing risks.
The Future of AI Ethics
Emerging Trends and Challenges
As AI technologies evolve, new ethical challenges will arise, such as ensuring fairness in generative AI models and managing autonomous systems’ societal impact.
Innovations in Ethical AI Practices
Advances in tools for bias detection, model interpretability, and fairness assessments will enable more ethical AI development.
Collaboration for Global Ethical Standards
International cooperation is vital for establishing universally accepted ethical guidelines. Initiatives like UNESCO’s AI Ethics Recommendation are steps toward this goal.
Conclusion
Ethical AI is critical for building trust and ensuring the responsible use of technology. By prioritizing transparency, fairness, accountability, and safety, organizations can create AI systems that are not only innovative but also trustworthy. As AI continues to shape the future, embracing ethical practices will be essential for long-term success and societal benefit.